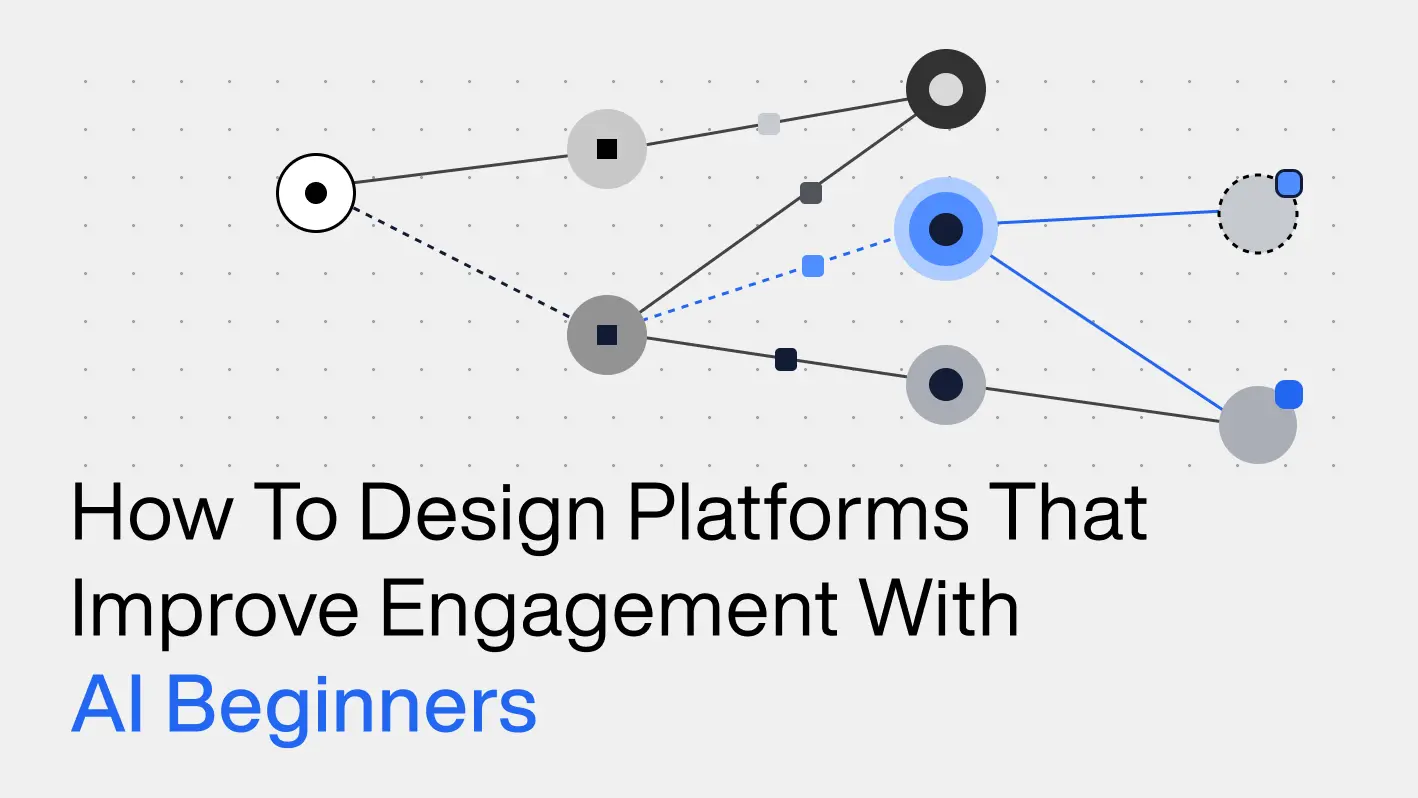
Artificial Intelligence (AI) has become a common element in enterprise-scale digital transformation efforts as it provides significant benefits to surfacing, monitoring, and predicting business insights across many industries. From operators and business analysts to data scientists and developers, users across all technical proficiency levels will — and already do — interact with AI daily. The diversity of this user range necessitates thoughtful design practices and user experiences to ensure the successful use and adoption of AI.
Designing AI for the User
At C3 AI, we have a diverse user base for our products from the more technically proficient, such as developers or data scientists, to the less technical, including business users across departments like sales, marketing, and operations. As we design products, we tend to think about our end users as AI personas in two main categories:
-
Novice AI users who are not as familiar with AI, such as sales representatives, teachers, and branding designers
-
AI-Savvy users who have more technical knowledge and experience, such as data scientists, data engineers, developers, and software engineers.
The design approach and best practices for these two audiences can be very different.
Take James, a sales representative, and Dana, a data scientist, as examples of these two disparate personas. James has not had much exposure to AI concepts or exercise model training. But as a salesperson, he understands key business processes and is a high-performing salesperson. Then, we have Dana, who has deep expertise in AI and works to enhance existing AI systems at her job.
This blog will cover how to design products for less technical and less AI-savvy users. Our next blog will discuss how to design products for technical, AI-savvy users.

James, our less AI-savvy friend

James has only had limited interactions with AI. He may imagine AI as a superpower or a black box. Because his relationship with AI is very limited, its overall sentiment and level of trust heavily relies on his first impressions of data performance. James might also have lower patience and higher barriers to understanding AI. However, he may still need to use it for his work as it can help fulfill his needs and alleviate his pain points.
Best Practices in Designing AI Products for Users Like James
1. Simplify and reduce the number of functions users need to learn
If you’ve ever tried to understand how data influences AI results, you might be overwhelmed by the amount of data, categories of metrics, and different ways data impacts a single output. For example, in figure 3 (below), you can see a set of metrics for our app designed for salespeople — the C3 AI CRM (Customer Relationship Management). Among those metrics, stock price is shown as impacting the win rate of a deal and is marked as High (-) in the column to the right. In other words, due to stock price changes, the possibility of a sales rep winning this deal has been impacted in a highly negative way.
However, to a salesperson like James, this might be too much information. For James, his goal was never to learn how AI works, but to quickly learn about useful signals affecting his work (e.g., stock price is reducing your win rate). To adjust the design to be friendlier to James (new design shown on the right side of figure 3), a more impactful way of presenting the information with just a down arrow next to stock price. This format lets the user know quickly that this factor is making a negative impact without any superfluous information like the level of a data impact score.

2. Provide relatable reference points for users
When we define the confidence level of AI, a common pattern is to offer an AI score that helps users sort through a list of items and prioritize them by importance or potential impact. However, if a user doesn’t have the context to understand an AI score, simply displaying this number can confuse users.
Let’s use the risk score as an example. To a user who understands this concept, risk scores can be helpful in determining how exactly they will use the suggested action given by the program — and if they want to take action at all. To an inexperienced user, a risk score of 88 could mean this action has a high risk, or it could be very safe — without training, any scoring system is arbitrary to a user. This means we must interpret that risk score for the user into a reference point they can understand without technical training:
“From a historical record, 80% of sensors with this risk score failed to complete their task.”
This information makes the score more trustworthy and helps users understand the severity of the situation more easily. Other helpful reference points include historical records, comparisons with average data, explanations for data severity (low, medium-high risk), and major factors/events that influenced the score.

3. Prioritize pertinent information and hide counter-intuitive data
Let’s look at one of our AI products that estimate property prices. Property appraisers use this product to price residential property that is going up for sale. During product development, specifically the model tuning process, our data scientists found that hundreds of factors contribute to a property’s price and we wanted to show all of those data points to property appraisers so they could assess the price from every angle.
However, we soon learned that this was too much information. Seeing every potential factor led users to feel lost and then eventually uncomfortable as they discovered that internal furnishing (i.e., home décor or staging) doesn’t contribute directly to a property’s market price. This led them to start questioning the model’s capabilities and their trust in it waned.
To these users, their years of experience were much more trustworthy than a new AI software showing data that contradicted what they considered deep knowledge of factors that affect property prices and the real estate market. And by displaying that data, we lost trust with the user.
When presented with a sea of information, people tend to find a familiar data point and determine if it information aligns with their own experience.
Now, we prioritize information based on its contribution to the model output. Instead of showing everything, we only show completely necessary pieces of information. And only when necessary, do we also provide them with the capability of digging deeper to see more or potentially all of the data points.
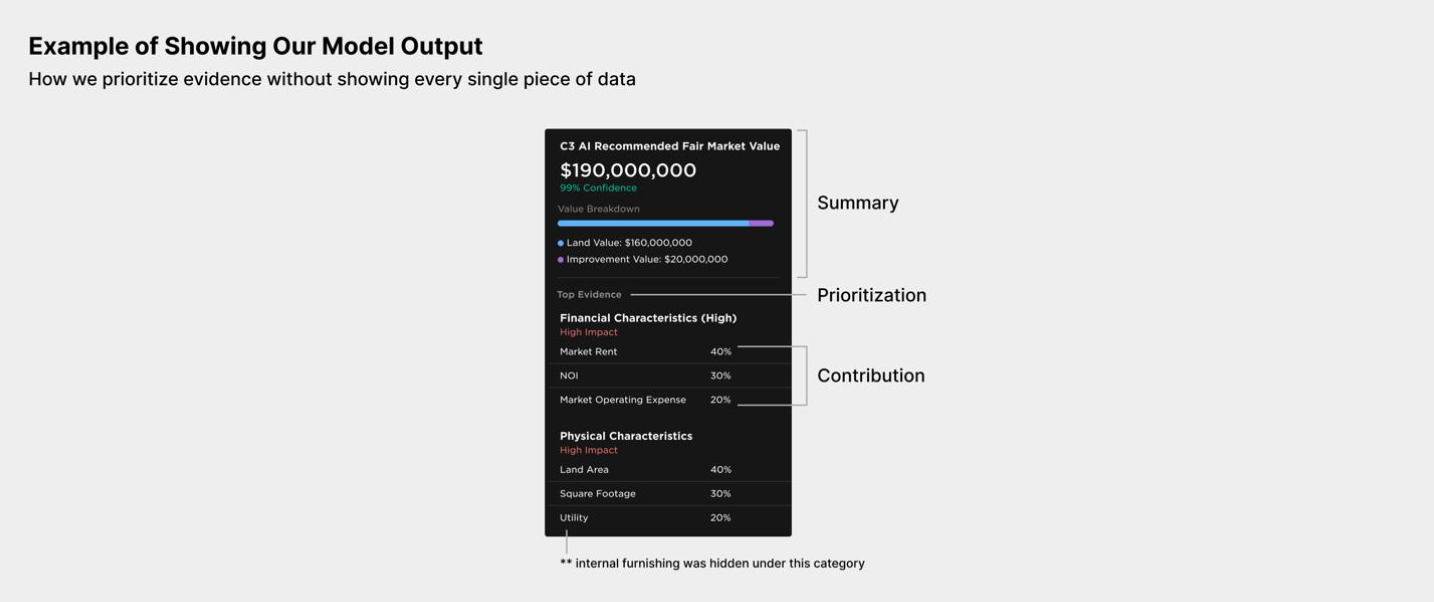
Key takeaways
-
Provide clear visual cues that don’t require users to interpret a message.
-
Give users context and interpret numbers (e.g., scores, data points, percentages) for them.
-
Prioritize key information and reduce noise for users.
Be sure to come back to check out our next blog that will take a look at how to design products for people who are very familiar with AI.
About the authors
Clair Sun is a product designer at C3 AI, where she leads design for products that are useful within the financial and supply chain industries. As indicated by her double degree in art and human-computer interaction from Carnegie Mellon University, Clair looks for well-designed, immersive experiences in galleries and museums. Prior to joining C3 AI, she worked as a designer at Deloitte Consulting.
Tianyi Xie is a senior product designer at C3 AI, where she leads product design for C3 AI CRM. She has a master’s degree in Interactive telecommunication from New York University and a bachelor’s degree in graphic design from Rhode Island School of Design. Prior to joining C3 AI, she worked on inclusive design and a11y (accessibility) projects for Google Files and Google Pay apps.